According to an article by KPMG entitled “Trusted Analytics Matter to CEOs”, nearly 50 percent of CEOs expect technological disruption to affect businesses in the next few years. They also believe that investment in D&A will help them respond to these challenges.
While they anticipate that D&A will help transform businesses, increase resilience, and enhance customer engagement, many are deeply concerned about the quality of data they use –they don’t trust it. KPMG suggests that developing this trust means evaluating data quality and integrity and to make sure analytics work as intended.
Importance of Trust
Unless D&A can be trusted, CEOs will make decisions based on instinct and judgement. Christian Rast, head of global D&A at KPMG says trust is essential for the development of mutually beneficial relationships. Business leaders know trust is fundamental for building meaningful relationships with customers, suppliers and within the organization. To be able to leverage data and analytics to guide their decision making, they must trust the information D&A provides.
Why CEOs Don’t Trust Their Data
According to KPMG, 56 percent of CEOs have concerns regarding integrity of their data. When the software they use to guide decision making is working with untrustworthy data, they naturally doubt its recommendations. Furthermore, they question the validity of the decision-making process because they don’t understand the software. For many CEOs, analytical algorithms are regarded as opaque black boxes and few understand the logical processes used to arrive at a recommendation.
Developing Trust in Analytics
Because the effective use of data and analytics presents so many advantages to businesses, it’s essential this lack of trust is overcome. This can only come about through a systematic approach that tackles each area of concern. According to KPMG, four elements of trust need to be addressed, and these are:
- Quality: The quality of data and how it’s used is of paramount importance. Data must be high-quality, valid, and sourced appropriately. Analytical methodologies should be thorough, accurate and applicable. Data collection and use must line up with best practices and industry standards.
- Effectiveness: It’s essential to verify that analytic processes produce valid solutions. Although it’s difficult to validate outcomes in complex scenarios, it must be done. One solution is the use of business models to predict known outcomes and to measure the degree to which they reflect what actually happens. Models should represent the whole organization and not just silos.
- Integrity: The way data is collected should comply with regulatory requirements and respect ethics and individual privacy. There should be transparency in the way data is collected and stored. Customers should know what data is collected and how it’s used–and that their right to privacy is respected.
- Resilience: Analytics solutions should be designed for the long term. They should adapt or be adaptable for changing situations. Regular testing should take place to ensure outcomes are predictable. Access to data should be carefully controlled to avoid breaches or leaks that could erode trust and confidence.
Overcoming Mistrust
According to KPMG, establishing trust is a long-term process that should start at the basics. Your first steps should be to:
- Identify weak spots in current processes and address them
- Ensure everyone understands the purpose for collecting data and how it will be used
- Engage all stakeholders, especially across functional lines
- Build expertise, and slowly but surely build understanding, competence and ultimately trust
Focusing on the RIGHT Data
While KPMG’s suggestions are spot on from a consulting perspective, there’s more to it than what is listed above. A major problem with data these days is that it’s pulled from disparate systems that have inconsistencies (both due to human interaction and the nature of how each system collects data). It’s near impossible (and completely unnecessary) to fix all of your data problems, so the first step is knowing where to start. Once you’ve brought the team together, focus on the most pressing business problems facing your company. The answer to this question should drive the kind of data you need to collect/clean.
In our line of work, we see far too many companies trying to collect as much data as possible. Inevitably, we see companies then thinking “well, we have all of this data, but it’s not good enough yet to do anything with.” This is only half correct. It’s impossible to know exactly what can be done with the data you have until you put it into a system that can a) tell you what data you need to address your key business problems and b) tell you whether the data you have is actually good enough to answer those questions. Customers are always shocked to find that they’re actually more ready than they think to start driving real business value with their data.
This is one of the many benefits of River Logic. Because you’re required to build a model relevant to your business/problem, you’re able to focus on the data you need. Further, with out-of-the-box model validation, the system will only provide an answer on “good” data. If there are inconsistencies or errors, River Logic will automatically find and point to those problems, enabling you to focus on fixing only the issues that 100% need to be addressed.
So, in addition to KPMG’s suggestions, here our a few more to think about. These might be carried out by your team, a company like River Logic, a consulting firm or some combination of those.
- Start with your most pressing business problems (2 or 3)
- Understand your “low-hanging fruit.” If you have or think you have the data — clean or not — begin your journey there
- Work with a team to understand what data you’ll need to address the problem, and develop a model of your business or business unit
- Add your data and validate the model to ensure all inconsistencies in data are addressed (this doesn’t take long, and it helps you focus on only relevant data)
Over time, this process will help build trust in your plans and help CEOs trust not only your data but also the process, insights and decisions you make.
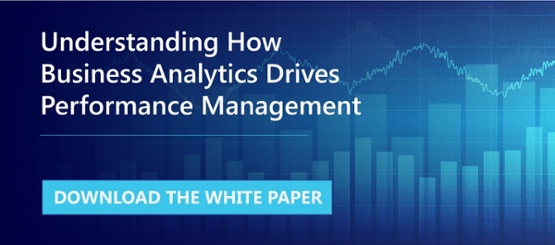