To illustrate the point, a recent paper from Ventana Research on supply chain planning revealed issues that companies using spreadsheet planning have experienced, including:
- 58% had difficulty managing supply chain processes
- Only 47% had accurate supply chain plans
- 53% had no ability to evaluate trade-offs
- Spreadsheets were reinforcing functional silos
- Collaboration was difficult
From this, it’s clear that relying on spreadsheets for planning is a costly exercise that hinders your ability to be competitive.
This may be the right time to abandon spreadsheets and switch to advanced analytics to improve supply chain planning and decision-making capabilities.
What Do We Mean by Advanced Supply Chain Analytics?
There are several kinds of supply chain analytics. Most are familiar with historical analytics, such as sales ratios, inventory turnover, number of stockouts and the like. These analytics, often called business intelligence, provide insight into past performance. While important, this information alone is not actionable; what’s needed are forward-looking analytics, better known as advanced analytics.
Advanced analytics allows you to predict what will happen with surprising accuracy. They also let you determine answers to questions such as what are the required stock levels needed to ensure business objectives are met and how to optimize revenue.
Predictive analytics leverage organizational data to predict future trends, while prescriptive analytics answers what-if questions to determine the best (optimal) supply chain planning decisions. This actionable information helps you remain competitive.
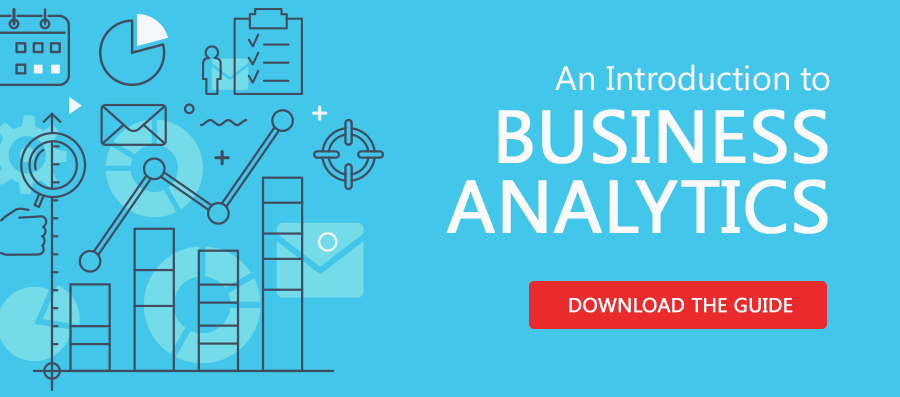
Supply Chain Analytics Examples
Numerous examples exist demonstrating how you can use advanced analytics to further organizational goals. Here are six typical supply chain analytics examples:
- Capacity planning
- Advanced sales and operations planning
- Simulation and scenario analysis
- Optimization
- Demand shaping
- Digital planning twin
Capacity Planning:
Capacity planning allows you to match procurement and manufacturing capacity to sales demand. It includes various strategies such as:
- A lead strategy that refers to installing capacity or producing goods in anticipation of demand
- A lag strategy where capacity is added once the current plant can’t meet demand
- The match strategy that involves incrementally adjusting capacity adjustments to match demand
Each approach has its merits, but the dilemma is knowing which is best for any particular situation. The answer lies in you developing a thorough understanding of your market, your competitors and those factors that affect demand.
While this may seem a tall order, it’s entirely possible using prescriptive analytics. The way it works is that you first develop a mathematical model of your business using the tools provided by a prescriptive analytics platform. Then, using powerful solver software to analyze your data, you can determine the right answers to questions such as what type of capacity planning is best for your business.
Advanced S&OP
Advanced sales and operation planning, sometimes called integrated business planning (IBP) is a next-generation S&OP that includes finance. Instead of the conventional S&OP focus on how to maximize widget production, advanced S&OP looks at the financial bottom line to determine the most profitable production and sales scenarios.
Additionally, it’s possible to close the loop between sales and production using a prescriptive analytics model that simultaneously models inputs and outputs, allowing you to determine the most feasible S&OP plan. This form of modeling can replace cumbersome manual S&OP processes that sequentially consider sales performance, sales forecasts, demand and procurement, a process that often takes weeks.
Because the prescriptive analytics model represents how the organization functions, it’s also possible to consider constraints and trade-offs and, using optimization capabilities, to establish the most profitable S&OP process strategy.
Simulation and Scenario Analysis
One of the crucial features of strategic planning is determining potential business scenarios and devising appropriate strategies. Royal Dutch Shell was one of the first major corporations to practice full-scale simulation and scenario planning. This work has helped shape the company’s future and guide it through numerous crises.
While few organizations can afford the cost of the multidisciplinary team approach used by Shell, prescriptive analytics modeling allows them to achieve similar results. Using a form of stochastic modeling, where the output of each set of decisions can act as inputs for the next set, optimization-based scenario planning can run through numerous simulations and determine the optimal answers to multiple what-if supply chain questions.
Optimization
Optimization refers to the use of prescriptive analytics to determine the optimal solution to a business problem. For example, it’s extremely difficult and complex to determine the optimal inventory strategy for a company involved in omnichannel retailing. On the one hand, there’s the need to ensure brick-and-mortar stores have adequate bulk stocks, while on the other, the need to fulfill multiple single orders to customers all over the country.
The solution lies with inventory optimization using prescriptive analytics. The first step, as always with prescriptive analytics, is to accurately model the organization’s business. Once the model is validated to ensure it reflects current business realities along with the effects of external factors, powerful non-linear solver software determines the optimal inventory strategy to deal with the complex and conflicting demands posed by omnichannel retailing. Solutions will vary from one organization to another and, for example, may involve the use of last-mile distribution warehousing, shipping from retail stores or third-party shipping.
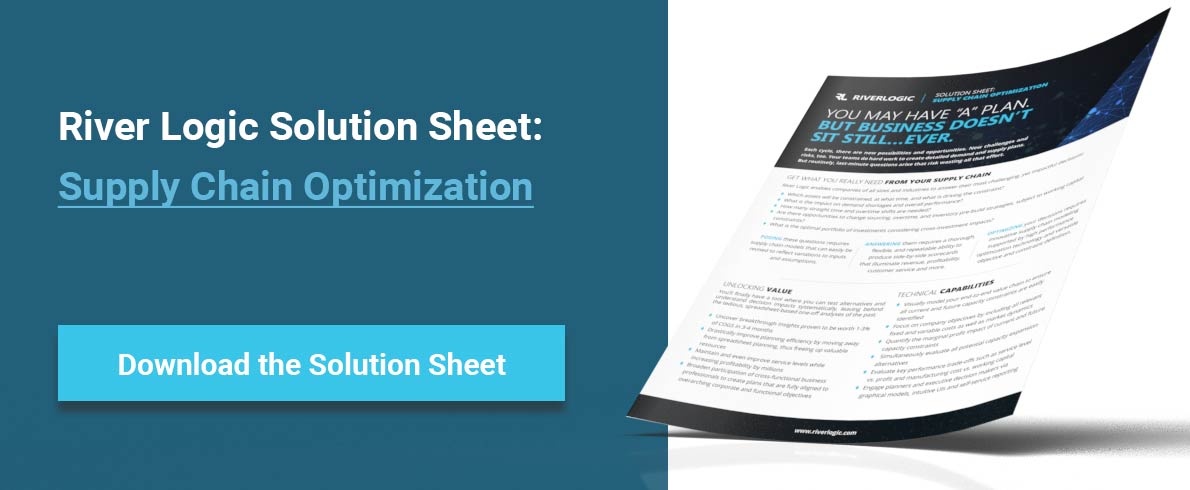
Demand Shaping
Demand shaping is what retailers apply to change demand for their products, rather than seeking to fulfill existing demand. A sales promotion is a typical example of demand shaping. The problem that often occurs is that sales promotions initiated by marketing don’t always correlate with procurement and manufacturing capabilities, the result being that a spike in demand causes stockouts and lost sales.
The answer takes both supply and demand into account. To do this, the model needs to be inclusive of sales, manufacturing and procurement as well as financials so that solutions are measured in financial terms. Then using what-if scenarios, it’s possible to determine the impact of a change in demand as a result of a sales promotion on the organization’s ability to meet the new demand and determine how to optimize supply and demand.
Digital Planning Twin
In all cases discussed, the first step is constructing a mathematical business model that accurately reflects how the organization functions. The next step, by uploading organizational data, is to solve various scenarios to determine the best course of action.
A digital planning twin takes this concept a step further in that the model is continuously updated with data from the real world. In this way, the model becomes dynamic, and it’s possible to use the model’s behavior to track what’s happening in the real world. The advantage is that it’s possible to react in real-time to unexpected developments and determine dynamic solutions as the need arises. River Logic’s SmartSolveâ„¢ is an example of an always available, scalable solution in the cloud that offers the prospect of real-time optimization.
Stay Competitive: Invest in the Right Form of Supply Chain Analytics
These six supply chain analytics examples illustrate how prescriptive analytics can solve multiple supply chain problems. Using prescriptive analytics, you create an accurate model of a part, or all, of your business. By populating this model with your data and evaluating different scenarios, it’s possible to solve your unique business challenges and gain a competitive edge.
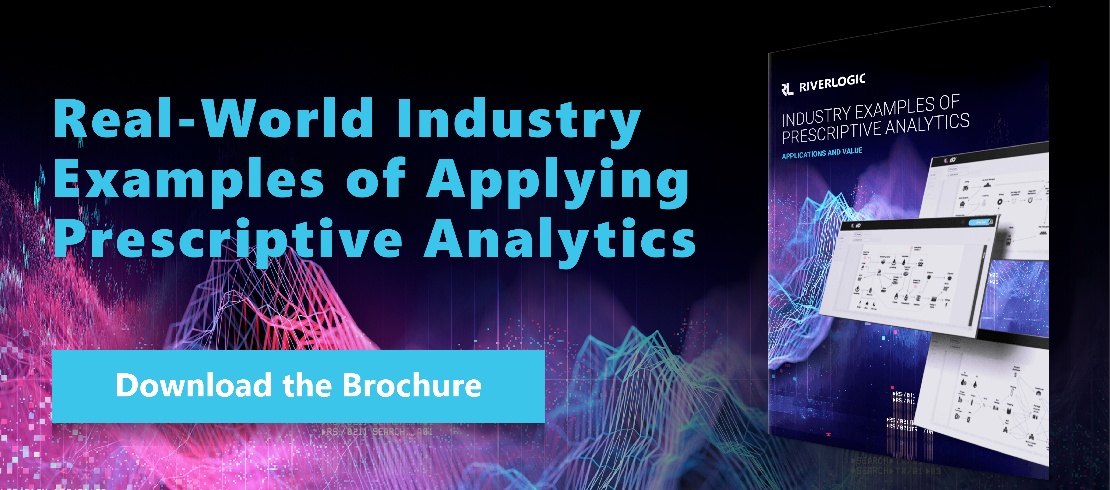