The problem centers on the ability of organizations to make effective use of the data held in their supply chain systems, and, at the same time, many are grappling with an almost exponential increase in the volume of this data. In their frustration, many supply chain managers initiate pilot projects to demonstrate the possibilities of supply chain analytics, but sadly, many of these never leave the laboratory. Other approaches, such as data mining, offer promise, but the time needed to develop effective solutions limits their attractiveness. Instead, numerous companies have discovered that an end-to-end approach supported by prescriptive analytics offers greater promise. Implementation is faster, which allows supply chain managers to make more high-impact, data-driven decisions.
Are You Getting Real Value from Your Data?
Relatively few businesses have succeeded in fully understanding all their data to actually grow their organization. In fact, in a 2018 survey of C-level technology and business executives reported in the Harvard Business Review, 72% of those surveyed said they didn’t even have a data culture. Reasons vary, but significantly:
- 77% felt that business adoption of big data initiatives was a significant challenge
- 93% said that barriers include cultural opposition from personnel and absence of business alignment
- Only 31% considered their organizations to be data-driven
Part of the problem is the explosion in the amount of data that companies hold. According to the IDC, the volume of data available in organizational supply chains has increased by more than a factor of 50 over the period between 2013 and 2018. Worryingly, less than 25% of that data is being used in data analysis in anything near to real time.
Dealing with the Exponential Growth in Supply Chain Data
The message is clear: Most organizations are not coping with the exponential growth of data. In the article in the Harvard Business Review, the authors reported a notable decline in the number of organizations using their data effectively and who considered themselves to be data-driven.
However, according to Gartner, the adoption of supply chain analytics represents the most effective way to handle this growth in supply chain data. Gartner believes effective implementation of supply chain analytics drives business growth. This view is supported by a survey of supply chain strategists conducted by Gartner, where nearly a third said they achieved a high return on their investment in analytics, while only 4% reported a negative return.
Why Traditional Supply Chain Analytics Aren’t the Answer
Traditional supply chain analytical solutions, often offered as part of a supply chain solution, tend to focus on past performance. These include sales reports, supplier compliance data, and other historic information. While this information is useful and necessary for measuring performance, it’s not directly actionable. Historical data highlights successes and failures and points where remedial action may be necessary, but it can’t answer the hard questions of what to do.
Similarly, predictive analytics that help to show what may happen in the future also lack the capability of directing operational decisions. Certainly, they identify the need to take action, but they can’t help executives decide which of a number of potential scenarios is best.
But this doesn’t mean that the lakes of structured and unstructured data can’t help determine the best way forward. Data mining, prescriptive analytics and other analytical tools can identify patterns in the data, and through the use of optimization processes, determine the best managerial decisions to preempt the future and grow the business.
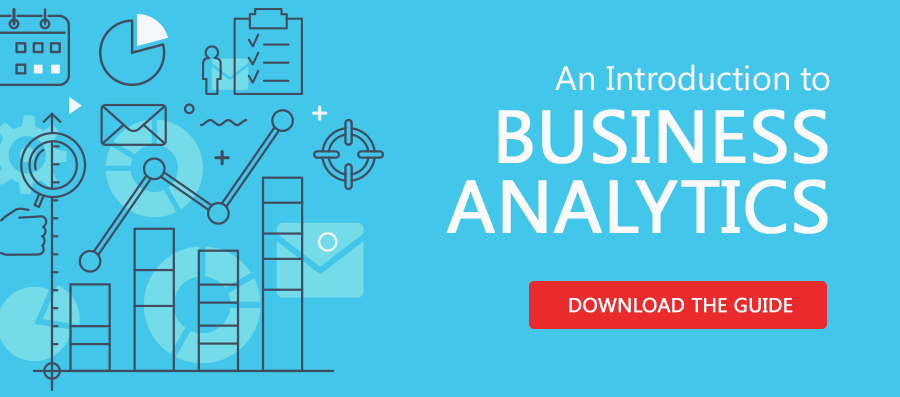
Is There a Place for Ad Hoc Supply Chain Analytic Solutions?
In the light of the fact that many CEOs and other executives are clearly skeptical about the efficacy of supply chain analytics, supply chain managers wanting to experiment with this concept may be tempted to consider ad hoc solutions. There’s clearly nothing wrong with this approach, although there are a number of pitfalls to consider.
Downsides of a Small-Scale Approach to Supply Chain Analytics Projects
Many small-scale projects flounder, especially those which aren’t formal pilot projects intended to evaluate full-scale supply chain analytics. This is partly due to the fact that it takes considerable resources to source, validate and blend data so it can be used. Additionally, organizational silos hinder access to information and limit their effectiveness. It’s estimated that 50% of small-scale data science projects never leave the laboratory.
Employing Data Scientists to Tackle Big Data Supply Chain Analytics
Part of the solution to cleaning up data is employing one or more data scientists with the requisite skills to organize and analyze data. Unfortunately, fully qualified data scientists are in short supply, and the field of potential applications is broader than the experience profile of many, especially in small organizations that can only afford one or two. Added to that is the fact that it takes considerable effort and time to develop hard-coded optimizing packages.
Packaged Supply Chain Analytics
A packaged supply chain analytics solution is one way of bypassing the need for a full-time data scientist. Packages usually incorporate software and tools needed to connect to and organize the data. Many have optimization tools allowing users to evaluate alternative scenarios. The main difficulty is matching the software solution to the business so the output is usable. For this reason, packages work better in well-defined businesses, such as retail and packaged goods.
Take the Bull by the Horns with a Holistic View of Supply Chain Analytics
Although small-scale projects can and do benefit the organization, a holistic approach is the only way to really get a handle on supply chain analytics. This is particularly in the light of best S&OP practice that involves sales, marketing and finance in planning processes. In order for supply chain analytics projects to succeed, they need support from the top along with full cooperation from other departments and especially from IT.
It needs to be appreciated that an effective supply chain analytics program entails a significant commitment of human and financial resources. At the same time, businesses that wholeheartedly invest in supply chain analytics find the return on investment is significant and well worth the implementation cost.
Maximizing the Value of Supply Chain Data with Prescriptive Analytics
While other forms of supply chain analytics present useful information, only prescriptive analytics offers the potential for supply chain managers to make informed, data-driven decisions. The reasons for this are two-fold.
Firstly, a real-time mathematical model of the business is prepared which accurately reflects supply chain realities. This model draws on the organization’s data, and it’s absolutely essential the model is validated against known data and outcomes. Secondly, optimization software is used to answer questions to known supply chain problems and issues. By accessing the organization’s data, plus other data streams such as supplier data, it’s possible to obtain specific answers to questions, such as how to increase profitability by reducing raw material and supply costs.
It’s through this type of approach to supply chain analytics that businesses can harness the information stored in their data to become more profitable and data driven.
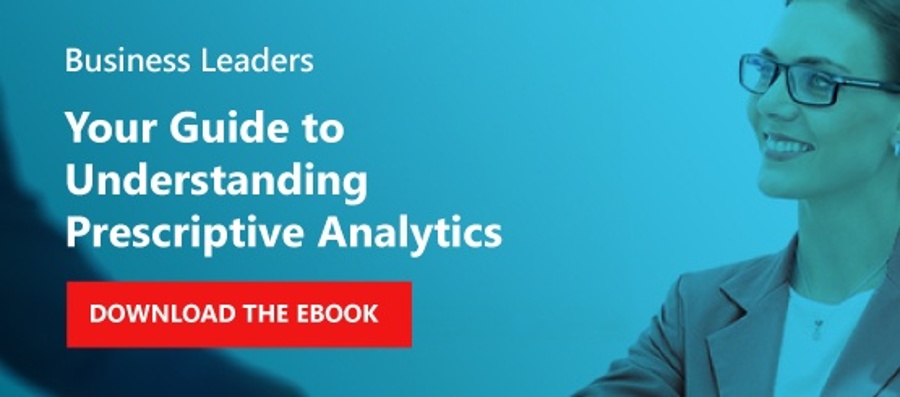